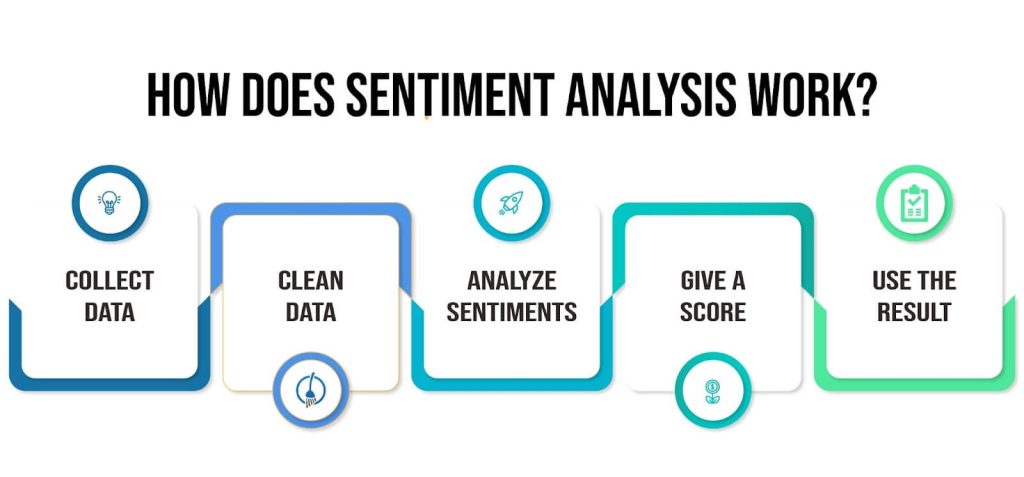
In the digital age, understanding public sentiment is crucial for businesses, marketers, and organizations. Sentiment analysis, often referred to as opinion mining, has emerged as a powerful tool to gauge public emotions and opinions. But how does it work? The answer lies in Natural Language Processing (NLP), a subset of artificial intelligence that enables machines to understand, interpret, and respond to human language. In this blog, we’ll explore how NLP pow77777ers sentiment analysis and why it matters.
What is Natural Language Processing (NLP)?
Natural Language Processing is a branch of AI that focuses on the interaction between computers and human language. It combines computational linguistics with machine learning algorithms to process and analyze large amounts of natural language data. NLP allows machines to perform tasks such as language translation, speech recognition, and, most importantly, sentiment analysis.
Understanding Sentiment Analysis
Sentiment analysis is the process of identifying and categorizing opinions expressed in a piece of text to determine whether the sentiment is positive, negative, or neutral. It is widely used in areas such as social media monitoring, customer feedback analysis, and market research. By analyzing the sentiment behind words, businesses can gain valuable insights into customer satisfaction, brand perception, and market trends.
The Role of NLP in Sentiment Analysis
NLP plays a critical role in sentiment analysis by enabling machines to understand the nuances of human language. Here’s how it works:
- Text Preprocessing:NLP begins with preprocessing the text, which involves tasks like tokenization (breaking text into words or phrases), stemming (reducing words to their root form), and removing stop words (common words like “and,” “the,” etc.). This step is essential to clean and standardize the data for analysis.
- Feature Extraction: NLP techniques extract features from the text that are relevant to sentiment analysis. These features could include word frequency, n-grams (combinations of words), and parts of speech. These features are then used to train machine learning models.
- Sentiment Classification: The core of sentiment analysis lies in classifying the text into positive, negative, or neutral categories. NLP uses machine learning algorithms like Naive Bayes, Support Vector Machines, or deep learning models to classify sentiment based on the features extracted from the text.
- Contextual Understanding: One of the biggest challenges in sentiment analysis is understanding context. NLP models are trained to recognize sarcasm, irony, and contextual nuances that can alter the sentiment of a statement. This is achieved through advanced techniques like word embeddings and transformer models like BERT (Bidirectional Encoder Representations from Transformers).
- Continuous Learning: As language evolves, so do sentiments. NLP models are continuously updated and trained on new data to improve accuracy and stay relevant. This continuous learning ensures that sentiment analysis remains effective over time.
Why Sentiment Analysis Matters
Sentiment analysis provides businesses with actionable insights that can drive decision-making. Here’s why it’s important:
- Customer Feedback: Analyzing customer reviews and feedback allows companies to understand their strengths and areas for improvement.
- Brand Monitoring: By tracking brand mentions on social media, businesses can gauge public perception and respond to potential crises.
- Market Research: Sentiment analysis helps companies understand market trends and consumer preferences, enabling them to tailor their products and services accordingly.
- Competitive Analysis: By analyzing the sentiment around competitors, businesses can identify opportunities and threats in the market.
Conclusion
Natural Language Processing is the backbone of sentiment analysis, enabling machines to decode human emotions hidden in text. As businesses continue to rely on data-driven insights, the role of NLP in sentiment analysis will only grow more significant. Whether it’s understanding customer feedback, monitoring brand reputation, or conducting market research, sentiment analysis powered by NLP provides the competitive edge needed in today’s digital landscape.
Read our latest blog:Top Trends Shaping Mobile UI/UX Design in 2024